The Use of Synthetic Data in Research – What Is It, Pros, Cons, and Risks
- Nashia Hussain
- 2 days ago
- 5 min read

What Is Synthetic Data?
Synthetic data is information that is artificially generated by computers rather than real-world events. The goal is to reproduce the patterns, relationships, and statistical properties of an original dataset without revealing any personal or sensitive information. For example, a synthetic dataset of patient records might demonstrate realistic correlations between symptoms and diagnoses, but none of the entries represent real individuals.
Key Benefits of Synthetic Data
Synthetic data offers numerous advantages, contributing to its growing adoption across various research fields.
Privacy Preservation is one of its key strengths, as it looks very similar to real data but does not contain any actual personal information. Hence, sensitive information such as patient health records, student data, and financial transactions can be used without compromising privacy.
Cost Reduction is another key advantage, as gathering and preparing real-world datasets can often be a time-consuming and costly process. It often involves conducting surveys, running experiments, or purchasing data from third-party sources. Once a synthetic data generation pipeline is set up, typically involving a combination of software tools that generate realistic, artificial data, this new data can be produced at a lower cost and easily expanded to meet growing needs.
Enhanced Data Diversity is another benefit of synthetic data, allowing researchers to overcome limitations or biases in real datasets. Since they can construct scenarios or data points that occur less frequently or may not occur at all in the original data.
Improved Data Sharing and Collaboration is an additional important benefit of synthetic data. Due to its negligible privacy or IP limitations, synthetic data can be shared more freely between researchers or even made public. For example, researchers from multiple hospitals can combine synthetic patient data sets and conduct a more statistically accurate study.
Challenges of Synthetic Data
While synthetic data offers many advantages, it also comes with important challenges and limitations that researchers must carefully consider:
Overfitting Risk occurs when models trained on synthetic data perform extremely well on that data but struggle to generalize to real-world inputs. This can happen if the synthetic data generator introduces its own biases or patterns that a model then learns too specifically.
Privacy Concerns are often overlooked, with many assuming that synthetic data is automatically safe to share. In reality, privacy is only guaranteed if the data is generated properly. If a generative model is poorly trained or overfits to the original data, synthetic records may identify real identities.
Regulatory Uncertainty remains a challenge, as synthetic data is still part of an emerging field where formal standards and regulations are still being developed. In fields like clinical trials or FDA approval processes, results based solely on synthetic data are not always accepted. As a result, researchers may face uncertainty over whether conclusions derived from synthetic data are accurate.
Technical Complexity is a common barrier, as developing a generative model that produces realistic data can be technically demanding and may require significant resources, including a steep upfront investment. Training Generative Adversarial Networks (GANs) or similar models can pose a technical challenge and usually requires tuning by an expert to produce realistic outputs.
Ethical Considerations
In addition to technical challenges, ethical concerns also have a significant role in the responsible application of synthetic data. Researchers and organizations should be concerned with the following:
Transparency is ethically important when working with synthetic data. Researchers should clearly communicate when synthetic data is being used and how it was generated. Stakeholders, whether other scientists, patients whose data was used to create a model, or the public, should not be misled into thinking synthetic data results are based on real observations when they are not.
Reproducibility can be strengthened through synthetic data, which simplifies data sharing across research teams. However, this benefit depends on whether the data is made accessible. When public sharing isn’t possible, creators should still provide access to legitimate researchers through agreements, similar to real data protocols, but with fewer privacy constraints.
Bias Mitigation is a key ethical consideration when working with synthetic data. Since one can generate more diverse data to balance out skewed real-world samples. On the other hand, if the process isn’t carefully managed, the data can perpetuate or even amplify biases present in the original data. Ethically, data scientists should audit synthetic datasets for representativeness.

Where Synthetic Data Works Best and Where It Doesn’t
Recommended Use Cases
Healthcare research and model development where patient privacy must be preserved.
Autonomous systems require simulated edge-case scenarios that are not easily captured in real-world data.
Financial services for testing models without exposing sensitive client information.
Education and social sciences, where strict data protection laws limit access to real datasets.
Examples Where Caution Is Needed
Regulatory submissions, such as clinical trials, where synthetic data is not accepted as standalone evidence.
Safety-critical environments (e.g., aviation, medical devices), where validated real-world data is required.
Legal and audit contexts, where only original records are considered valid for compliance.
Managing Synthetic Data Responsibly with myLaminin
Research data management platforms play a key role in enabling the responsible use of synthetic data.
Security and Access Control remain important, even when working with synthetic data. While privacy risks may be reduced, protections are still necessary. Role-based access control is essential, hence, myLaminin allows management of who can access which datasets or even which parts of a dataset. The platform can also restrict viewing of those to only authorized team members. myLaminin supports tagging data as PHI (Protected Health Information) and hiding or masking those fields for users who don’t have PHI clearance.
Compliance Automation helps streamline steps that would otherwise be cumbersome in the research process. For instance, a data-sharing agreement or NDA is needed before sharing any data, real or synthetic, between institutions. myLaminin streamlines this by automating the request and receipt of data-sharing agreements and consent forms through the platform.
Collaboration and Versioning are key strengths of synthetic data, especially when supported by the right platform. Instead of sending datasets over email or download links, myLaminin allows researchers to “publish” datasets to a secure portal where collaborators can access them. If a dataset is updated or a new synthetic version is generated, the platform can version it and notify all users.
Audit Trails and Traceability are essential when working with synthetic data. myLaminin addresses this by leveraging blockchain to create immutable audit logs. One can trace how a synthetic dataset was created, from which source data and when it was created. If any issues arise, for example, showing compliance with HIPAA or GDPR in case of inquiries, having this trace allows quick investigations and can demonstrate that proper procedures were followed.
Addressing GDPR and the 'Right to Be Forgotten'
A common question about synthetic data and compliance is whether it can meet laws like the General Data Protection Regulation’s (GDPR) “right to be forgotten,” also known as the "right to erasure". In some cases, synthetic data can help with this, especially when it is created in a way that completely separates it from any identifiable source records.
However, synthetic data cannot be considered a definitive replacement for formal anonymization. To be exempt from the EU’s GDPR regulations, the synthetic data must be created so that re-identification is impossible. If there is a possibility that the synthetic data could be traced back to a specific individual, it may still be subject to GDPR requirements.
Conclusion
Platforms like myLaminin give researchers the tools they need to manage synthetic data responsibly. That includes keeping data secure, tracking how it's used, and helping meet compliance requirements. Looking ahead, research is likely to take a hybrid approach using both real and synthetic data, where each is most effective.
References
__________________________________
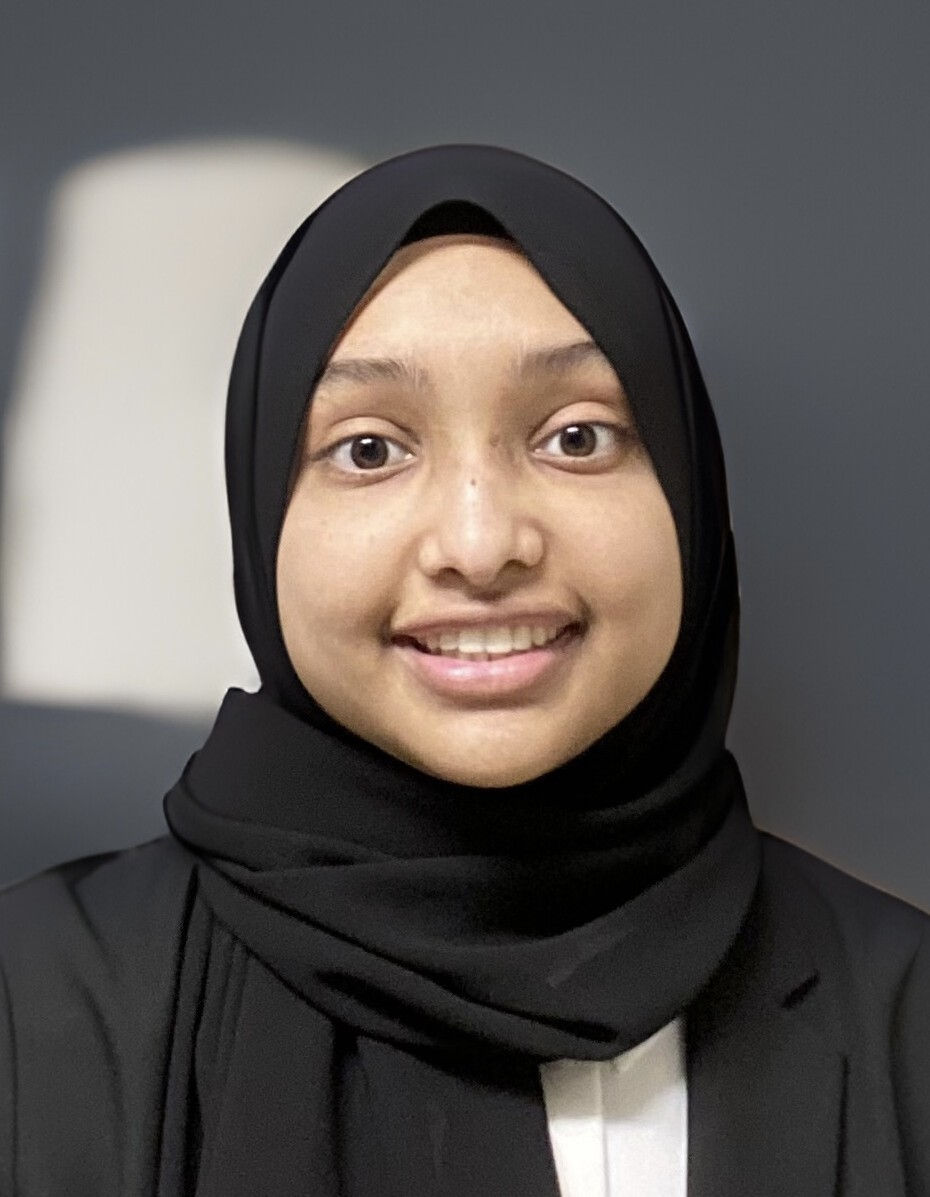
Nashia Hussain (article author) is a myLaminin intern studying Business Administration at York University, Schulich School of Business.
Comments